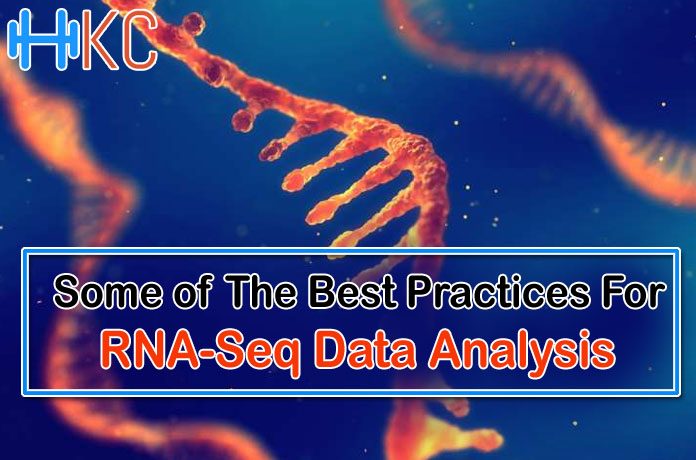
Some of The Best Practices For RNA-Seq Data Analysis
Today, the popularity of NGS platforms ensures that each team has several experimental options at hand. They can vary in terms of experiment design, library preparation, sequencing steps, and analysis platforms.
RNA sequencing experiments still bring forth unique challenges in experiment design and data analysis.
What influences the best practices of RNA Seq analysis?
The set of best practices for an RNA Seq analysis experiment varies significantly depending upon the goal of the investigation, the latest literature and specific steps that might be necessary for extending the application of an RNA Seq analysis experiment.
The first key to a successful RNA Seq analysis experiment is to have a good experimental design.
Understandably, qualitative RNA analyses and qualitative experiments will have different requirements. They can stem from differences in the starting RNA amount, the number of replicates, types of replicates, library preparation, sequencing platforms, coverage and depth, and read length.
The preprocessing and visualization of data
The raw data that sequencing machinery generates can provide the read counts or molecular counts. The existing raw-data processing and management pipelines typically take care of quality control, genome alignment, and quantification.
1. Reads processing and quality control
The RNA Seq analysis pipeline demands the presence of multiple checkpoints. The raw reads come in the FASTQ format that stores the ribonucleotide sequences juxtaposed to a per base quality score. The scores can range from 0 to 40. The first step of QC involves checking the volume of reads per sample, base qualities, and general read. It also considers the G+C content, PCR primers, unexpected repetitive sequences, and unclipped adapters in the sample. Preprocessing reads is crucial in ensuring a high-quality analysis of the RNA sequences. It is standard for the bases in the 3′ end to generate lower quality. Trimming may improve the mappability but shortening the sequences can increase the errors in mapping.
While modern mapping tools can take care of unaligned read ends, there is no consensus on which tool or platform is the best for mapping RNA reads. For RNA seq de novo assembly, you can leverage supplementary tools to ensure the best quality of reads by joining the overlapping paired-end reads.
Base error correction is another method that can replace trimming and filtering. It also increases the net volume of useful data and contig sizes.
2. Mapping and assembly of read sequences
After processing the raw reads, you can choose from a wide range of available approaches for mapping. The availability of a reference sequence should govern your choice. Your reads can be mapped to the genome. Its expression can be easily quantified. You can complete your transcriptome assembly beginning with the reference sequence. It can result in multiple contigs correlating to a particular gene and its functional isoforms. If the species is new and lacks a reference library, you can assemble the reads via de novo assembly.
A word on sequence mapping
Choosing the best mapping technology is no joke. The standard mapping tools like BLAST are better for traditional pair-wise alignment of contigs. You need a tool that can take care of the sequencing errors, sequence variability like SNP, and IN/DEL mutations. The best way to guarantee reproducibility of published results, you should mention the mapper tool used, allowed seed mismatches, treatment for multi-mapping reads, and minimal alignment score. Due to the wide range of algorithms and programming language each mapping tool uses, it is indeed impossible to find one that is the best one for all RNA sequencing experiments.
3. Visualization of data
The mapping data comes in SAM formats. These files are difficult to interpret and access the available text editors. You need a wholesome NGS platform that can help in the interpretation of the SAM files via the use of graphical browsers.
4. Downstream analysis
The downstream analysis of RNA seq data involves several steps –
a. Quantification and differential expression of genes
The goal of your RNA sequencing experiment can be to quantify the differential expression of a gene under variable conditions. Then, you need an NGS platform that can perform feature counts and HTSeq-count. A set of housekeeping genes with non-differential expression is necessary as the quality control of the experiment. RNA sequencing provides a precise estimate of RNA abundance in a given sample, but other techniques like quantitative PRC and real-time PCR are necessary for the validation of these finds.
b. Annotation
It refers to the identification of the location of the genomic elements. The RNA Seq analysis can provide sufficient data for determining the location of the genomic elements. They can improve the precision of existing annotations. One can only derive open reading frames (ORFs), tRNA, and rRNA from using genome sequences for annotation. Using BLAST is traditional in this step. However, annotation of multiple short reads within a small timeframe can be quite challenging on BLAST or BLASTX.
c. Alternative splicing
Alternative splicing (AS) is a ubiquitous post-transcriptional modification that increases the challenge of mapping a set of cDNA reads to a genome. Analysis of AS requires splice-aware platforms that can align transcripts to the reference genomes. AS demands the inclusion of an additional quality control step that can reconstruct the transcript keeping the splicing isoforms in mind. Again, due to the expansive range of software dependent upon multiple coding languages and algorithms, it is challenging to outline just one that is best for every experiment.
d. Fusion genes
These are chimeras that are common in tumor cell lines. They are important biomarkers. However, they can pose a significant challenge in RNA sequencing data analysis. If your RNA seq experiment aims to identify fusion genes, you need NGS technologies that can align transcripts with the corresponding region that may have undergone inversions, INDEL mutations, trans-splicing, or rearrangements.
The accuracy of the method depends upon high-quality read alignments that support disputatious mapping. The NGS method should also be able to facilitate paired-end or single-end sequencing.
The recent advances in NGS technology have fostered the growth of genomics studies. They have allowed the affordable generation of vital biological information. From genetic engineering to personalized medicine; RNA sequencing data analysis has facilitated the advancement of several fields of biological sciences.
However, the sheer variety of applications of RNA Seq analysis keeps us from zeroing in on a set of best practices that may benefit every researcher and scientist out there.